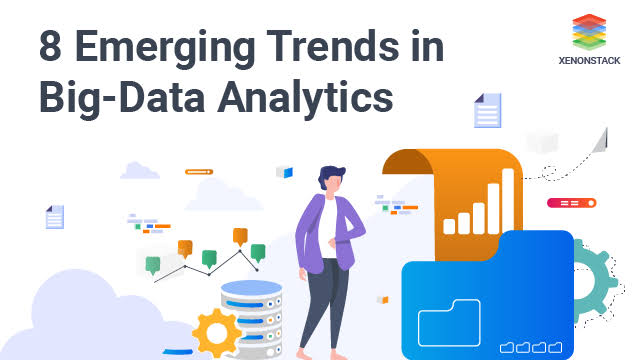
Top trends in Big Data analytics for 2022 and Beyond
You will be surprised by the fact that each day we are producing more data in 2 days than decades of history. Yes, that’s true, and most of us do not even realize this thing that we produce so much data just by browsing on the Internet. If you don’t want the future technologies to catch you off guard, pay attention to these current trends in big data analytics and succeed!
Trends in big data analytics
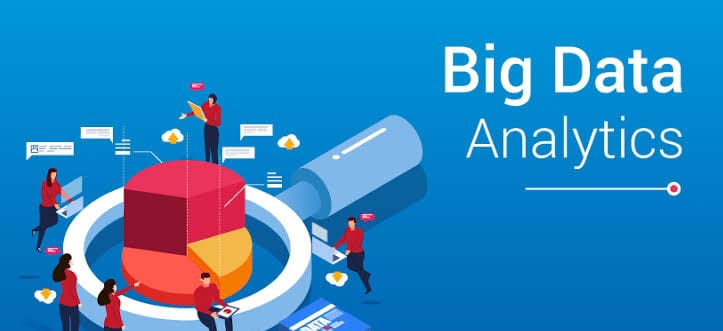
Analytics Prediction
Big data analytics has always been a critical component of a company’s strategy for gaining a competitive advantage and achieving its objectives. They employ fundamental analytics tools to prepare massive data and figure out what’s causing specific problems. Predictive methods are used to analyze current data and historical occurrences in order to better understand customers and identify potential threats and events for a company. Big data predictive analytics can foresee what will happen in the future. This method is quite effective at correcting studied data and predicting customer response. This allows businesses to outline the steps they need to take by predicting a customer’s next move before they take action.
Quantum Computing
Quantum Computing is a type of computing that uses quantum mechanics. Processing a large volume of data with present technology can take a long time. Quantum computers, on the other hand, calculate the probability of an object’s state or occurrence before measuring it, implying that they can process more data than conventional computers. We can drastically cut processing time by compressing billions of data at once in only a few minutes, allowing enterprises to make more rapid decisions and achieve more desired outcomes. Quantum computing may be able to help in this procedure. Quantum computers being used to rectify functional and analytical research across multiple firms could make the sector more exact.
Edge computing
Edge Processing is the process of running processes on a local system, such as a user’s computer, an IoT device, or a server. Edge computing moves computation to the network’s edge, reducing the amount of long-distance communication required between a consumer and a server, making it one of the most recent big data analytics developments. It boosts Data Streaming, including real-time data Streaming and processing while keeping latency to a minimum. It allows the devices to reply very instantly. Edge computing is a cost-effective approach to handle large amounts of data while using less bandwidth. It can help a business cut development costs and make software run in faraway places.
Hybrid Clouds
An on-premises private cloud and a third-party public cloud are combined in a cloud computing system, which is orchestrated through two interfaces. By transferring operations between private and public clouds, hybrid cloud offers greater flexibility and additional data deployment options. To achieve adaptability with the targeted public cloud, a company must have a private cloud. It must build a data center, which includes servers, storage, a LAN, and a load balancer, in order to accomplish this. To support the VMs and containers, the company must implement a virtualization layer/hypervisor. Also, a private cloud software layer should be installed. Data can be transferred between private and public clouds thanks to the use of software.
Data as service
Traditionally, data has been stored in data stores designed to be accessed by certain applications. Daas was just the beginning when SaaS (software as a service) became widespread. Data as a service, like Software-as-a-Service applications, makes use of cloud technology to provide users and apps with on-demand access to information regardless of their location. One of the current developments in big data analytics is Data as a Service, which will make it easier for analysts to get data for business review activities and for areas around a company or industry to exchange data.
Natural Language Processing (NLP)
Natural Language Processing (NLP) is a branch of artificial intelligence that focuses on improving computer-human communication. NLP’s goal is to read and decode human language. Natural language processing is based on machine learning and is used to create word processors and translation software. Algorithms are needed in Natural Language Processing Techniques to recognize and extract the necessary data from each sentence using grammatical rules. Natural language processing primarily employs syntactic and semantic analysis techniques. Sentences and grammatical difficulties are handled by syntactic analysis, whereas the meaning of the data/text is handled by semantic analysis.
Artificial Intelligence that is both responsible and smart
Better learning algorithms with a shorter time to market will be enabled by responsible and scalable AI. AI technology will help businesses do much more, such as creating effective processes. Businesses will figure out how to scale AI, which has been a difficult task thus far.
XOps
XOps (data, machine learning, model, platform) is a method of achieving efficiencies and economies of scale. DevOps best practices are used to achieve XOps. As a result, efficiency, reusability, and repeatability are ensured while technology, process replication, and automation are reduced. With flexible design and agile orchestration of controlled systems, these improvements would enable prototypes to be scaled.