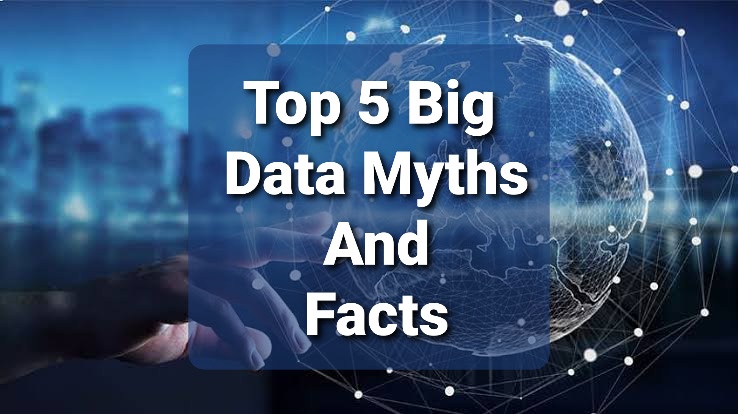
Top 5 Big Data Myths and Facts
Businesses deal with an abundance of data on a daily basis and the volume of data being created and stored is an ever-increasing phenomenon, which needs scrutiny and analysis. This is where the concept of big data analytics comes in.
Big data uses data points from a set of raw data and identifies relationships to gain new insights and make predictions about the future. The raw set of data is used to build models, run simulations and alter data points to see how each alteration impacts the resulting information/insight and prediction. This has been adopted by organizations to aid with smart and more informed decisions.
Big Data does, in fact, hold a lot of potential for all businesses, regardless of their size. An organization can gain insights from big data analysis that help them not only boost revenue but also recognize the gaps in their service and goods.
Let’s take a look at some of the most common Big Data myths and facts.
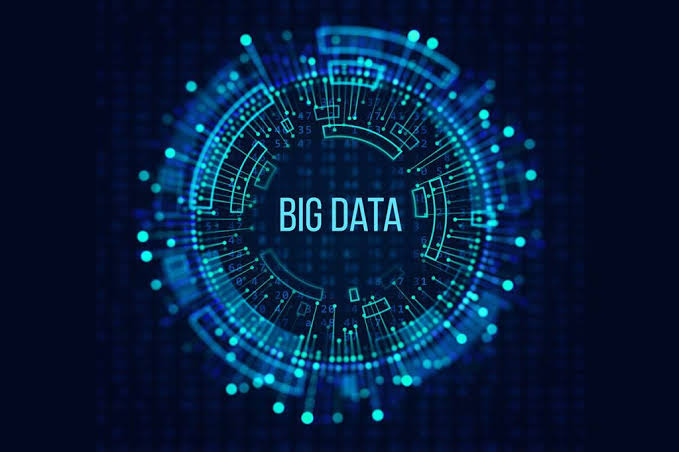
Big data has a few fallacies because it is a developing technology and a relatively new notion. However, if we don’t assess. Big data myths against accurate Big data facts, we risk making blunders.
As a result, we will address the common Big data myths and the accompanying Big data realities in this blog so that you can learn the truths. This will assist you in debunking Big Data fallacies and ensuring that your business runs smoothly.
Myth 1: Big data is all over the place.
Fact: It is true that Big Data technology and services are currently the focus of industry interest, with record-high adoption. However, according to Gartner Big data facts and figures, just 73 percent of all enterprises are planning and investing in Big data. They are, however, still in the early stages of Big Data implementation.
Surprisingly, only 13% of the companies polled had implemented big data solutions. The primary issues that organizations face, according to Gartner big data facts, are how to get value from Big data with correct strategy.
Furthermore, because it is a complicated technology, many firms face challenges during the pilot stage because they do not link the technology to a specific application.
Myth 2: Big Data requires a large budget and is only for large organizations.
Fact: It is true that large-scale data centers and high-end technology for integrating Big data have been built by organizations such as multinational firms and government agencies. Not only that, but due to a scarcity of resources in the market, hiring skilled big data specialists and data scientists is also quite expensive.
But, alas, the time is passed. As Big data’s utility has grown, vendors such as Apache have cut the cost of licensing Big data technologies, making them more affordable. They’re also introducing new tools and strategies to help organizations dig through data collection.
Myth 3: The concept of machine learning is linked to Big Data.
Fact: Machine learning frequently deals with large amounts of data. The core principle of machine learning, on the other hand, is to use such data to model the underlying process in order to make it more efficient. Furthermore, machine learning is exclusively dependent on machine learning algorithms that can interpret large data sets and then use what they’ve learned to make useful conclusions.
As a result, combining Big and Machine learning can yield significant insights.
Myth 4: Big data eliminates the need for a data warehouse.
Fact: First and foremost, a data warehouse is an architecture, whereas big data is solely a technological endeavor. As a result, one cannot technically replace the other. Big data is a technology that saves and manages massive amounts of data in order to use it for various Big data solutions at a fair cost.
Data warehouses, on the other hand, organize data as a framework to deliver a single version of it. It combines data from several sources and organizes it in an accessible format. It also offers a data lineage capability, which aids in determining the data’s origin.
Myth 5: Big Data is always of good quality.
Fact: Big data does not always imply clean and high-quality data. Big data, on the other hand, frequently contains data quality issues. Furthermore, cleaning huge data is required in order to gain better and more accurate insights from it. As a result, it is a mistake to believe that there is no need for data cleansing, collection, or analysis of Big data.